Evolution of Smart Factory Data Analytics
Smart factory data analytics have undergone a significant evolution in recent years, shaping the way manufacturing processes are managed and optimized. Initially, smart factories relied heavily on descriptive analytics, which involved the use of historical data to understand past performance and trends within the manufacturing environment. While descriptive analytics provided valuable insights, there were limitations in terms of its ability to uncover the root causes of issues and predict future outcomes accurately.
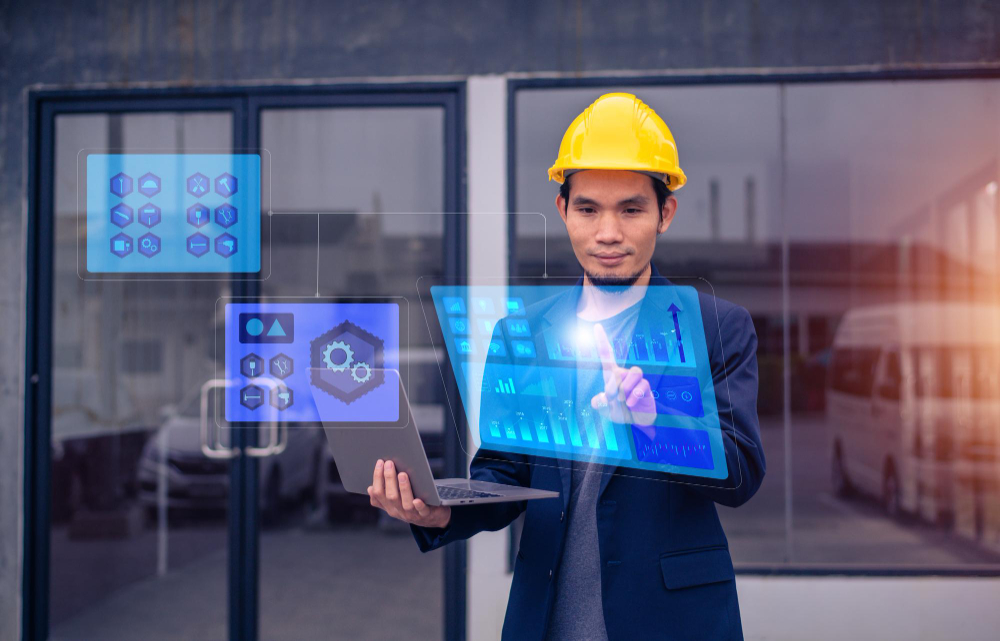
The transition to diagnostic analytics marked a crucial shift in smart factory data analytics, as manufacturers began to investigate why certain events occurred within their operations. By leveraging advanced analytics techniques, such as root cause analysis and statistical modeling, diagnostic analytics enabled manufacturers to gain a deeper understanding of the factors influencing their performance. This shift towards a more proactive and investigative approach laid the foundation for the adoption of predictive analytics in smart factories, paving the way for more informed decision-making and enhanced operational efficiency.
• Diagnostic analytics allowed manufacturers to investigate why certain events occurred within their operations
• Leveraged advanced analytics techniques such as root cause analysis and statistical modeling
• Enabled a deeper understanding of the factors influencing performance
• Shift towards a more proactive and investigative approach in smart factory data analytics
The adoption of predictive analytics in smart factories represented a significant leap forward in the evolution of data analytics within manufacturing environments. By utilizing machine learning algorithms and artificial intelligence, predictive analytics enabled manufacturers to forecast future outcomes with greater accuracy and identify potential issues before they escalated. This shift towards predictive capabilities empowered manufacturers to make data-driven decisions in real-time, optimize production processes, reduce downtime, and ultimately enhance overall productivity.
• Predictive analytics utilized machine learning algorithms and artificial intelligence
• Forecasted future outcomes with greater accuracy
• Identified potential issues before they escalated
• Empowered manufacturers to make data-driven decisions in real-time
Looking ahead, the next phase of evolution for smart factory data analytics is prescriptive analytics. This advanced form of analytics not only predicts what will happen but also suggests actions that can be taken to optimize outcomes based on various scenarios. By combining historical data, real-time information from sensors and IoT devices, along with external factors such as market trends or supplier performance, prescriptive analytics has the potential to revolutionize decision-making processes within smart factories. With prescriptive insights at their disposal, manufacturers can proactively address challenges, capitalize on opportunities, and drive continuous improvement across all facets of their operations.
• Prescriptive analytics not only predicts what will happen but also suggests actions for optimization
• Combines historical data with real-time information from sensors/IoT devices & external factors
• Has the potential to revolutionize decision-making processes within smart factories
Proactively addresses challenges & drives continuous improvement across operations
Descriptive Analytics in Smart Factories
Descriptive analytics in smart factories involve the gathering and analysis of historical data to understand past performance and trends. By examining historical data, companies can gain insights into various aspects of their operations, such as production output, machine maintenance, and energy consumption. This helps manufacturers identify patterns and make informed decisions based on past performance, leading to improved efficiency and productivity.
One key aspect of descriptive analytics is the use of data visualization tools to present complex data in a clear and understandable format. Visualizations such as graphs, charts, and heat maps enable factory managers to quickly interpret data and identify areas that require attention or improvement. This visual representation of data fosters better communication and collaboration among teams, facilitating more effective decision-making processes in smart factories.
Challenges with Descriptive Analytics
Challenges may arise in the realm of Descriptive Analytics within smart factories due to limitations in providing deeper insights beyond historical data summaries. The reliance on past information might hinder the ability to uncover underlying patterns or causality, potentially impeding proactive decision-making processes. Furthermore, the static nature of Descriptive Analytics can restrict businesses from adapting swiftly to dynamic operational changes within the manufacturing environment.
In addition, the lack of predictive capabilities within Descriptive Analytics poses a challenge for smart factories aiming to enhance efficiency and optimize production processes. Without the ability to forecast potential outcomes or trends, organizations may struggle to pre-emptively address issues before they escalate, leading to inefficiencies and missed opportunities for improvement. As the landscape of smart factory data analytics evolves, overcoming these challenges with Descriptive Analytics becomes crucial for driving innovation and remaining competitive in the rapidly advancing industry.
Transition to Diagnostic Analytics
Diagnostic analytics marks a crucial stage in the evolution of smart factory data analytics. This phase involves a deeper dive into the data collected from various sources within the factory floor to identify patterns, anomalies, and root causes of issues. By leveraging advanced analytics tools and techniques, businesses can move beyond simply describing what is happening in their operations to understanding why it is happening.
Transitioning to diagnostic analytics empowers organizations to make more informed decisions based on a clearer understanding of their manufacturing processes. By uncovering the underlying reasons behind performance bottlenecks, quality issues, or equipment failures, companies can proactively address these challenges and optimize their operations. This shift towards a more diagnostic approach sets the stage for further advancements in predictive and prescriptive analytics, driving continuous improvement and innovation within smart factories.
Benefits of Diagnostic Analytics in Smart Factories
Diagnostic analytics in smart factories offer a range of advantages to businesses striving for operational excellence. By analyzing historical data and identifying patterns, companies can uncover inefficiencies and issues in real-time, allowing for proactive decision-making and timely interventions. This proactive approach enables smart factories to optimize production processes, minimize downtime, and ultimately improve overall productivity.
Moreover, diagnostic analytics provide valuable insights into the root causes of performance bottlenecks and operational challenges. By understanding these underlying issues, organizations can implement targeted solutions to enhance efficiency, reduce costs, and enhance quality control measures. Armed with actionable data-driven insights, smart factories can drive continuous improvement initiatives and stay ahead of the curve in a rapidly evolving manufacturing landscape.
Moving towards Predictive Analytics
As smart factories continue to advance, there is a notable shift towards predictive analytics in the realm of data analysis. This transition marks a pivotal moment in the evolution of smart manufacturing, enabling companies to anticipate potential issues and opportunities before they arise. By leveraging historical data, machine learning algorithms, and real-time insights, predictive analytics empowers organizations to make proactive decisions that optimize operations and enhance overall efficiency.
The implementation of predictive analytics in smart factories brings about a myriad of benefits. From minimizing unplanned downtime and improving asset management to boosting quality control and streamlining production processes, the predictive capabilities offer a competitive edge in the increasingly dynamic manufacturing landscape. Through harnessing the power of predictive analytics, companies are better equipped to adapt to changing market demands, enhance productivity, and drive sustainable growth in the rapidly evolving industrial sector.
Implementing Predictive Analytics in Smart Factories
When it comes to implementing predictive analytics in smart factories, companies are leveraging advanced technologies to anticipate potential issues and make proactive decisions. By utilizing historical data, real-time information, and machine learning algorithms, predictive analytics can help identify patterns and trends that may indicate upcoming equipment failures or production delays. This proactive approach allows manufacturers to optimize their processes, reduce downtime, and improve overall efficiency within the factory environment.
Furthermore, predictive analytics in smart factories enables predictive maintenance strategies, where maintenance tasks are performed based on data-driven predictions rather than fixed schedules. This shift towards predictive maintenance not only minimizes unexpected breakdowns but also extends the lifespan of machinery and equipment. As a result, companies can save costs on unnecessary repairs, enhance equipment reliability, and ensure continuous production operations to meet customer demands.
Advantages of Predictive Analytics
Predictive analytics in smart factories offer a proactive approach to addressing potential issues before they arise. By leveraging historical data and advanced algorithms, predictive analytics can forecast equipment failures, production downtimes, and quality control issues. This capability enables manufacturers to take preemptive measures, such as conducting preventive maintenance or adjusting production schedules, to minimize disruptions and optimize operational efficiency.
Furthermore, predictive analytics empower smart factory managers to make data-driven decisions based on accurate forecasts rather than reactive responses. This predictive capability allows for better resource allocation, improved inventory management, and enhanced overall production planning. By harnessing the power of predictive analytics, smart factories can achieve higher levels of productivity, reduced costs, and increased competitiveness in today’s rapidly evolving manufacturing landscape.
The Shift to Prescriptive Analytics
Prescriptive analytics marks a significant advancement in smart factory data analysis, providing actionable insights that go beyond predicting outcomes to recommending optimal solutions. By harnessing the power of data, prescriptive analytics empowers decision-makers with recommendations that drive efficiency, improve processes, and maximize productivity within smart factories. This transformative approach enables organizations to proactively address potential issues before they arise, leading to better resource allocation, enhanced performance, and ultimately, increased competitive advantage in the rapidly evolving industrial landscape.
Implementing prescriptive analytics in smart factories requires a strategic integration of advanced technologies, including machine learning, artificial intelligence, and digital twin simulations. These tools work in tandem to analyze vast amounts of real-time data, identify patterns, and predict future outcomes with a high degree of accuracy. By leveraging prescriptive analytics, manufacturers can streamline operations, optimize supply chain management, and fine-tune production processes to meet dynamic market demands effectively. The shift to prescriptive analytics signals a paradigm shift in data-driven decision-making, setting the stage for a new era of agility, innovation, and sustainable growth in smart manufacturing environments.
Utilizing Prescriptive Analytics in Smart Factories
Prescriptive analytics in smart factories leverages advanced technologies and algorithms to recommend optimal courses of action. By analyzing historical data and current conditions in real-time, prescriptive analytics can provide actionable insights to enhance decision-making processes. This proactive approach allows smart factories to anticipate potential issues and identify opportunities for improvement before they occur, leading to increased efficiency and productivity.
Implementing prescriptive analytics in smart factories requires a robust data infrastructure and integration with existing systems. By combining data from multiple sources and applying machine learning algorithms, manufacturers can gain valuable insights into their operations and make informed decisions to drive continuous improvement. The ability to automate recommendations based on data analysis empowers organizations to optimize processes, reduce costs, and stay ahead of market trends in today’s competitive landscape.
Benefits of Prescriptive Analytics
Prescriptive analytics in smart factories offer a strategic advantage by providing actionable insights that guide decision-making processes. By utilizing advanced algorithms and machine learning techniques, prescriptive analytics helps optimize production processes, minimize downtime, and enhance overall operational efficiency. This leads to improved resource allocation, reduced waste, and increased profitability for manufacturing organizations.
Furthermore, prescriptive analytics enables real-time adjustments and recommendations based on changing variables, enhancing agility and responsiveness within smart factory environments. By predicting potential issues before they occur and suggesting optimal solutions, prescriptive analytics empowers companies to proactively address challenges and make informed choices for sustainable growth and competitive advantage in the rapidly evolving manufacturing landscape.
Future Trends in Smart Factory Data Analytics
As smart factory data analytics continue to advance, one of the future trends to watch out for is the integration of artificial intelligence (AI) and machine learning algorithms. These technologies will enable smart factories to make more accurate predictions and decisions in real-time, ultimately improving operational efficiency and reducing downtime. Additionally, the incorporation of Internet of Things (IoT) devices and sensors will provide even more data points for analysis, leading to a more comprehensive understanding of the manufacturing processes.
Another emerging trend in smart factory data analytics is the emphasis on cybersecurity measures to protect sensitive data and intellectual property. With the increasing interconnectedness of devices and systems in smart factories, the risk of cyber threats is also escalating. Implementing robust security protocols and encryption methods will be crucial in safeguarding valuable information and maintaining the integrity of operations in smart factories.
Key Takeaways from the Evolution of Smart Factory Data Analytics
In the evolution of smart factory data analytics, it is evident that the shift from descriptive analytics to diagnostic analytics has enabled manufacturers to move beyond simply understanding what has happened to identifying why it occurred. This transition has paved the way for more proactive decision-making and problem-solving within smart factories, ultimately leading to enhanced operational efficiency and productivity.
Furthermore, the progression towards predictive analytics signifies a significant advancement in leveraging data to anticipate future events and trends. By utilizing predictive models and algorithms, smart factories can not only forecast potential issues but also optimize processes and resources to preemptively address them. This shift towards a more proactive and data-driven approach is reshaping the manufacturing landscape, paving the way for increased competitiveness and sustainability in the industry.
Additional Resources:
[catlist categorypage=”yes”]
Table of Contents
Categories:
[categories orderby=name]
Latest Posts:
[sbs_latest_posts]
FAQs:
What is the role of descriptive analytics in smart factories?
Descriptive analytics helps in summarizing historical data to provide insights into what has happened in the past in a smart factory setting.
What are some challenges with descriptive analytics in smart factories?
Some challenges include limited data sources, data silos, and the inability to provide deeper insights or predictive capabilities.
How does diagnostic analytics differ from descriptive analytics in smart factories?
Diagnostic analytics goes a step further by identifying the root causes of issues or trends seen in the descriptive analytics phase.
What are the benefits of implementing diagnostic analytics in smart factories?
Diagnostic analytics can help in understanding the reasons behind certain patterns or trends, leading to more informed decision-making and quicker problem resolution.
What is predictive analytics and how is it used in smart factories?
Predictive analytics uses historical data to predict future outcomes or trends in a smart factory setting, enabling proactive decision-making and maintenance.
What are some advantages of implementing predictive analytics in smart factories?
Advantages include reduced downtime, optimized production schedules, improved product quality, and overall cost savings.
How does prescriptive analytics differ from predictive analytics in smart factories?
Prescriptive analytics not only predicts future outcomes but also provides recommendations on how to optimize processes or make improvements based on those predictions.
How can prescriptive analytics be utilized in smart factories?
Prescriptive analytics can be used to optimize production schedules, maintenance routines, resource allocation, and other operational decisions in a smart factory setting.
What are some benefits of utilizing prescriptive analytics in smart factories?
Benefits include increased efficiency, reduced waste, improved decision-making, and overall optimization of processes in a smart factory environment.
What are some future trends in smart factory data analytics?
Future trends may include the integration of AI and machine learning, real-time analytics, IoT-enabled analytics, and greater emphasis on cybersecurity measures.
What are the key takeaways from the evolution of smart factory data analytics?
Key takeaways include the importance of moving from descriptive to prescriptive analytics, leveraging predictive capabilities for proactive decision-making, and staying abreast of emerging trends to stay competitive in the smart factory landscape.