The Evolution of Manufacturing Technology
Manufacturing technology has undergone a significant transformation over the years, driven by advancements in automation, robotics, and digitalization. Traditional manufacturing processes that relied heavily on manual labor have been replaced by sophisticated machines and computer-controlled systems. This shift towards automation has not only improved production efficiency but has also led to higher levels of precision and consistency in manufacturing operations.
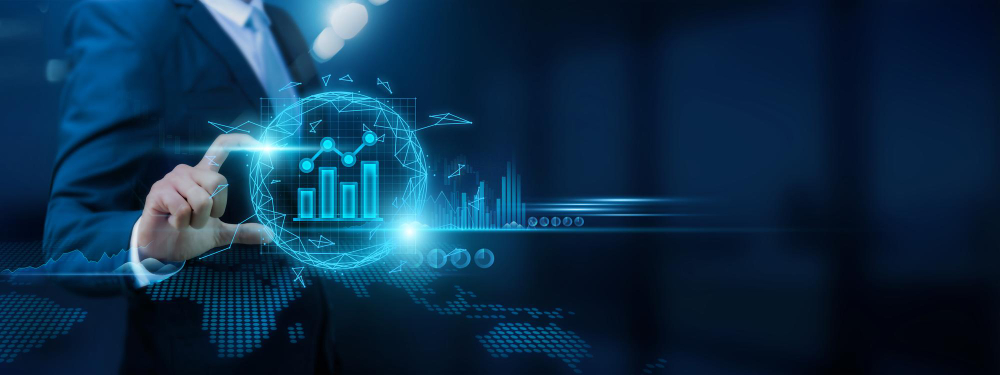
Furthermore, the integration of technologies such as the Internet of Things (IoT) and artificial intelligence (AI) in manufacturing has paved the way for smart factories. These interconnected systems can communicate and analyze data in real-time, enabling manufacturers to make data-driven decisions and optimize their production processes. As manufacturing technology continues to evolve, the industry is poised for further innovation and growth in the coming years.
Understanding the Role of Data in Smart Factories
Data plays a crucial role in the operations of smart factories, enabling manufacturers to extract valuable insights that drive performance improvements and operational efficiency. By leveraging data generated from various sensors, machines, and production processes, manufacturers can gain real-time visibility into their operations, identify bottlenecks, and optimize production workflows. This data-driven approach allows for predictive maintenance scheduling, better resource allocation, and overall cost reductions.
Moreover, data analytics in smart factories facilitates the implementation of quality control measures by detecting defects or anomalies in the production process early on. This proactive approach not only helps in minimizing waste and rework but also ensures that products meet the desired quality standards before they reach the customers. With the power of data at their fingertips, manufacturers can make informed decisions, streamline their processes, and stay competitive in the rapidly evolving manufacturing landscape.
• Data plays a crucial role in the operations of smart factories
• Manufacturers can gain real-time visibility into their operations by leveraging data from sensors, machines, and production processes
• Predictive maintenance scheduling, better resource allocation, and cost reductions are possible through data-driven approaches
• Data analytics in smart factories helps implement quality control measures by detecting defects early on
• Minimizing waste and rework while ensuring products meet desired quality standards is facilitated by proactive use of data
• Informed decision-making, streamlined processes, and competitiveness are enhanced with the power of data in manufacturing.
Benefits of Implementing Analytics in Manufacturing
One of the primary advantages of implementing analytics in manufacturing is the ability to gain valuable insights into operational efficiency. By analyzing data collected from various processes within the factory, organizations can identify areas for improvement and optimize their production line. This data-driven approach enables manufacturers to make informed decisions that result in cost savings and increased productivity.
Another benefit of utilizing analytics in manufacturing is the enhancement of quality control measures. By monitoring key performance indicators and analyzing real-time data, companies can detect potential issues early on and take corrective actions to maintain product quality standards. This proactive approach not only reduces the likelihood of defects but also improves customer satisfaction and brand reputation.
Challenges of Utilizing Big Data in Smart Factories
The implementation of big data analytics in smart factories comes with its own set of challenges. One major obstacle is the sheer volume of data being generated by the various sensors and connected devices on the factory floor. Managing and processing this massive amount of data in real-time can strain existing infrastructure and lead to bottlenecks in the system. Additionally, ensuring data quality and accuracy is crucial for making informed decisions, but with the sheer volume of data being collected, there is a risk of encountering inaccuracies or inconsistencies in the data.
Another challenge in utilizing big data in smart factories is the need for skilled personnel who can interpret and analyze the data effectively. Data scientists and analytics experts are often required to extract meaningful insights from the data and translate them into actionable strategies for optimizing production processes. However, there is a shortage of skilled professionals in this field, making it difficult for companies to fully leverage the potential of big data in their manufacturing operations.
Key Metrics to Measure in Smart Factory Analytics
In smart factory analytics, there are key metrics that play a crucial role in assessing performance and driving improvements. Overall Equipment Effectiveness (OEE) is a fundamental metric that combines availability, performance, and quality to provide a comprehensive view of manufacturing efficiency. By measuring OEE, manufacturers can identify areas for optimization and enhance overall productivity.
Another essential metric in smart factory analytics is the First Pass Yield (FPY), which measures the proportion of products manufactured correctly the first time without any rework or repair. FPY is a critical indicator of production quality and operational efficiency. By monitoring and analyzing FPY, manufacturers can pinpoint quality issues early on, reduce waste, and ensure customer satisfaction.
The Impact of Real-Time Data Analysis on Production Efficiency
Real-time data analysis plays a crucial role in enhancing production efficiency within smart factories. By continuously monitoring and analyzing data streams from various sources, manufacturers can make informed decisions promptly. This immediate access to insights enables real-time adjustments to production processes, leading to improved productivity and reduced downtime. With the ability to quickly identify and address bottlenecks or inefficiencies, manufacturers can optimize their operations for maximum output.
Furthermore, real-time data analysis allows for predictive maintenance practices to be implemented effectively. By leveraging machine learning algorithms and historical data, manufacturers can anticipate equipment failures before they occur, enabling proactive maintenance to prevent costly breakdowns. This proactive approach not only reduces maintenance costs but also minimizes unplanned downtime, ultimately contributing to increased production efficiency and operational reliability in smart factories.
Optimizing Supply Chain Management with Smart Factory Analytics
Supply chain management plays a crucial role in the success of manufacturing operations, impacting various aspects such as production efficiency, inventory management, and timely delivery of products. With the integration of smart factory analytics, manufacturers gain valuable insights into their supply chain processes, enabling them to optimize sourcing, production scheduling, and distribution strategies. By leveraging real-time data and analytics, organizations can proactively identify bottlenecks, forecast demand accurately, and streamline their supply chain operations for improved cost efficiency and customer satisfaction.
Smart factory analytics also facilitate enhanced visibility and transparency throughout the supply chain network, allowing manufacturers to monitor key performance indicators, track supplier performance, and identify potential risks or disruptions in advance. Through predictive analytics and machine learning algorithms, companies can optimize inventory levels, reduce lead times, and enhance collaboration with suppliers and logistics partners. By harnessing the power of data-driven insights, organizations can make informed decisions, mitigate supply chain complexities, and ultimately achieve a competitive advantage in today’s dynamic manufacturing landscape.
Predictive Maintenance and Machine Learning in Manufacturing
Predictive maintenance and machine learning are revolutionizing the manufacturing industry by enabling proactive equipment maintenance based on data-driven insights. By analyzing historical data and real-time performance metrics, companies can predict when machines are likely to fail and take preemptive actions to avoid costly downtime. This shift from reactive to proactive maintenance strategies not only increases operational efficiency but also reduces maintenance costs significantly.
Machine learning algorithms play a crucial role in predictive maintenance by identifying patterns and anomalies in equipment behavior that human operators may overlook. These algorithms can analyze vast amounts of data to uncover hidden correlations and predict potential equipment failures with a high level of accuracy. By harnessing the power of machine learning, manufacturers can optimize maintenance schedules, extend equipment lifespan, and ensure continuous production operations with minimal disruptions.
Enhancing Quality Control through Data-Driven Insights
In today’s manufacturing landscape, quality control plays a pivotal role in ensuring the production of flawless products. Leveraging data-driven insights has proven to be a game-changer in enhancing quality control processes within smart factories. By harnessing real-time data analytics, manufacturers can gain valuable visibility into their operations, identifying potential defects or issues at an early stage and taking corrective actions swiftly. This proactive approach not only minimizes the risk of faulty products reaching customers but also helps in optimizing overall production efficiency.
Furthermore, data-driven insights provide manufacturers with a deeper understanding of the root causes behind quality deviations, enabling them to implement targeted solutions and preventive measures. By analyzing a multitude of data points related to production processes, equipment performance, and product quality metrics, smart factories can identify patterns, trends, and anomalies that impact quality control. This knowledge empowers manufacturers to make informed decisions, fine-tune their processes, and continuously improve product quality standards.
Innovative Technologies Driving the Future of Smart Factories
Smart factories are being revolutionized by a host of innovative technologies that are driving the industry towards unprecedented levels of efficiency and productivity. One of the key advancements is the Internet of Things (IoT), which enables seamless connectivity and data sharing between machines, devices, and systems in the manufacturing environment. Through IoT integration, smart factories can collect real-time data to optimize processes, predict maintenance needs, and enhance overall operational performance.
Another crucial technology shaping the future of smart factories is artificial intelligence (AI) and machine learning. These technologies empower machines to analyze vast amounts of data, detect patterns, and make autonomous decisions to improve manufacturing processes. AI-driven predictive maintenance, quality control systems, and production planning tools are streamlining operations and reducing downtime, leading to significant cost savings and enhanced competitiveness in the global market.
Case Studies on Successful Implementation of Analytics in Manufacturing
In one case study, a multinational automotive manufacturer successfully implemented analytics in their production processes to optimize efficiency and reduce downtime. By collecting and analyzing real-time data from their equipment and machinery, the company was able to identify potential issues before they escalated, leading to significant cost savings and improved overall productivity. The integration of predictive maintenance algorithms enabled the manufacturer to schedule maintenance proactively, preventing unexpected breakdowns and minimizing production disruptions.
Another notable case study involves a leading electronics manufacturer that leveraged data analytics to enhance quality control and defect detection in their assembly lines. By analyzing data generated from sensors and cameras installed in key production stages, the company was able to identify patterns and anomalies that indicated potential defects early in the manufacturing process. This proactive approach not only improved the overall quality of their products but also reduced waste and rework, ultimately boosting customer satisfaction and brand reputation.
Best Practices for Integrating Data Analytics into Smart Factory Operations
When integrating data analytics into smart factory operations, it is crucial to start by clearly defining the objectives and key performance indicators (KPIs) that will drive the analytics strategy. This involves identifying the specific areas where data analytics can bring the most value, such as improving production efficiency, optimizing maintenance schedules, or enhancing quality control processes. By aligning analytics initiatives with the overall business goals of the smart factory, organizations can ensure that their efforts are focused and impactful.
Another best practice is to invest in the right technology and tools that can support the collection, storage, analysis, and visualization of data. This may include implementing a robust data management system, utilizing advanced analytics software, or deploying IoT devices to capture real-time data from machinery and equipment. By leveraging the latest technologies, smart factories can streamline their data analytics processes and uncover actionable insights that drive continuous improvement and innovation.
The Future Outlook of Smart Factory Analytics in Industry 4.0
As we look ahead to the future of smart factory analytics in Industry 4.0, it is evident that the integration of advanced technologies such as artificial intelligence, machine learning, and Internet of Things (IoT) will play a pivotal role in shaping the manufacturing landscape. These technologies will enable real-time data collection, analysis, and decision-making, ultimately leading to more efficient and agile production processes. Moreover, the use of predictive analytics and digital twins will further enhance operational performance by anticipating maintenance needs and simulating production scenarios for optimal results.
With the increasing complexity of global supply chains and the rise of customer expectations for personalized products, smart factory analytics will be instrumental in driving innovation and competitiveness in the manufacturing sector. By harnessing the power of big data and analytics, manufacturers can gain deeper insights into their operations, identify patterns and trends, and make data-driven decisions to improve overall efficiency and quality. The future outlook for smart factory analytics in Industry 4.0 is one of continuous evolution and adaptation to meet the changing demands of a rapidly transforming industry landscape.
Additional Resources
[catlist categorypage=”yes”]
Table of Contents
Categories:
[categories orderby=name]
Latest Posts:
[sbs_latest_posts]
FAQs:
What is Industry 4.0?
Industry 4.0 refers to the current trend of automation and data exchange in manufacturing technologies. It includes cyber-physical systems, the Internet of Things, cloud computing, and cognitive computing.
How can smart factory analytics benefit manufacturers?
Smart factory analytics can provide manufacturers with valuable insights to optimize production processes, improve efficiency, reduce costs, and enhance product quality.
What are some challenges of utilizing big data in smart factories?
Challenges of utilizing big data in smart factories include data security concerns, data integration issues, the need for skilled data analysts, and the complexity of managing and analyzing large volumes of data.
How can predictive maintenance and machine learning benefit manufacturing?
Predictive maintenance and machine learning can help manufacturers anticipate equipment failures, reduce downtime, improve maintenance scheduling, and ultimately increase productivity.
What are some key metrics to measure in smart factory analytics?
Key metrics to measure in smart factory analytics include production output, machine downtime, quality control metrics, supply chain performance, and overall equipment efficiency.
What are the best practices for integrating data analytics into smart factory operations?
Best practices for integrating data analytics into smart factory operations include setting clear objectives, investing in the right technology and tools, training employees on data analytics, and regularly monitoring and evaluating performance.
How can real-time data analysis impact production efficiency in smart factories?
Real-time data analysis can help manufacturers quickly identify and address production issues, optimize workflow processes, make informed decisions, and improve overall production efficiency.